At the present moment, AI researchers are studying new form and architecture to develop the future AI. Today the main approaches and form are symbolic (based on representations of problems, logic and search) Bayesian networks (probabilistic model) and deep learning. Most people are familiar with a deep learning application that involved an explicit notion of modularity and subroutines, such as Neural Module Networks or Neural Programming Interpreters like, Google Translate (GT) that uses a large end-to-end long short-term memory network in which the system “learns from millions of examples”.
Deep learning powers our phone’s face unlock feature. If it weren’t for deep learning, Spotify and Netflix wouldn’t have any recall on what you want to hear or watch next.
However, is it realistic in the future to have different architecture to capture cognition? Or what will be the architecture of future be for AI?
To explore these questions the Montreal Institute for Learning Algorithms organizes in December a debate with two renowned researchers: Gary Marcus, best-seller author, entrepreneur and Yoshua Bengio, University of Montreal professor, laureate of the 2019 Turing Award (Nobel Prize of Computing) and Deep Learning expert.
During the debate both Gary Marcus and Yoshua Bengio agree that the field of AI might benefit from an articulation of their agreements and disagreements. They agreed that allowing humans to interactively train artificial agents to understand language instructions is important for both practical and scientific reasons.
Today there is no current learning methods to train artificial intelligence agents (AI) to understand human language instructions. Marcus and Bengio agreed that this goal may require substantial research efforts. They expose research and various models to develop strategies to improve data efficiencies of language learning.
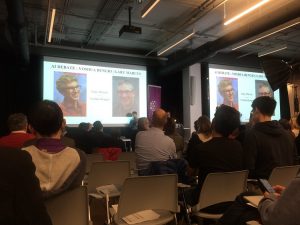
Bengio and Marcus Debate Which is the way to go forwards with AI?
According to Bengio, deep learning has made a lot of progress in perception and one of its main ambitions is to design algorithms that learn better representations. Representations that should be connected to the high-level concepts that we use in language, but this is not something we know how to do with unsupervised learning yet.
“To really understand a sentence in natural language, you need have a system which understands what the words in the sentence refers to in real world, with images, sounds and perception. One more idea in the prior consciousness is to ground those high-level concepts and rules in low-level perceptions. The research directions go to grounded language learning and multimodal language models, where the learning is not just texts but also their associations with images, videos and sensory perceptions,” said Bengio. (M)
Gary Marcus has a different approach and he is searching for a form that would involve causality and logic. “Realistically, deep learning is only part of the larger challenge of building intelligent machines. Such techniques lack ways of representing causal relationships (…) have no obvious ways of performing logical inferences, and they are also still a long way from integrating abstract knowledge, such as information about what objects are, what they are for, and how they are typically used.” (Wikipedia)
During the debate Bengio explain briefly his new research which involve learning representations of high-level concepts. It would be a kind of concept manipulate with language and integrating abstract knowledge. Bengio called this model Prior consciousness.
After the debate, I did some research to better comprehend the relation between deep learning and Prior consciousness with human cognition.
Deep learning and Prior consciousness in relation to human cognition
Deep learning is closely related to a class of theories of brain development (specifically, neocortical development) proposed by cognitive neuroscientists in the early 1990s. A variety of approaches have been used to investigate the plausibility of deep learning models from a neurobiological perspective. Although a systematic comparison between the human brain organization and the neuronal encoding in deep networks has not yet been established, several analogies have been reported. For example, the computations performed by deep learning units could be similar to those of actual neurons and neural populations. Similarly, the representations developed by deep learning models are similar to those measured in the primate visual system both at the single-unit and at the population levels. (Wikipedia)
Bengio prior to consciousness model is related to the consciousness global workspace theory. Bengio approach is base on the idea of an incentive regulator for representations and to force them to have the property that we can extract just a few dimensions at a time and to make powerful predictions about the future. It’s a way to add an extra constraint to learning representations so that they will be good at expressing classical AI symbolic knowledge. The question Bengio was asking during the debate was about “maintaining attention”. Per say, how would be possible for a learning agent to continuously learn, adjust the mapping while enabling higher levels to retain their understating of the lower level.
In his research he gave the example ; “consider predicting whether a pile of blocks will fall on or off a table. It involves a high-level discrete outcome which can be predicted easily, even if the details of where the blocks will fall is very difficult even for humans to predict. In that case, predicting the future at the pixel level would be extremely difficult because future states have high entropy, with a highly multi-modal distribution. However, some aspects of the future may have low entropy. If in addition, these aspects have a big impact on predicting what will come next (or on taking the right decisions now), then the consciousness prior should be very useful.” (Bengio, 2017)
According to my study on the mechanism of the consciousness and the sensation of presence and immersion felt by users interacting in virtual space (Farrell, 2008), researchers would have everything to gain if they studies the exceptional work of Mario Robriguez Cobos (Silo). Cobos works on the activity of the consciousness explained that the image is an active form, that place the consciousness (as structure) in-the-world. In the psychology of the image, Cobos describe how the image act on the body and the “body-in-the-world” because of intentionality, which is directed outside itself and does not simply correspond to a for-itself or some “natural,” reflected, and mechanical in-itself. The image acts within a temporo-spatial structure and within an internal “spatiality” that has thus been termed the “space of representation.” The various and complex functions that the image carries out depend in general on the position it occupies within that spatiality.
In the work contribution to thought Cobos (Silo) give a fuller justification the theory of the space of representation. His work explore also the fundamental mechanism of the consciousness and the emplacement of attention in higher level of prediction. Cobos called it the reversibility of the consciousness which is the ability of the consciousness to direct itself, by means of the attention, to the sources of information. Thus, for the senses, the reversible mechanisms result in what we he called apperception, and for the memory, they result in evocation. The operation of the reversible mechanisms is directly related to the level of consciousness; as one ascends in level they function more, and as one descends they function less. Of course Cobos concepts and theory require an understanding of the theory of consciousness describe in the psychology of the new humanism.
About Deep learning
Deep learning is a friendly facet of machine learning that lets AI sort through data and information in a manner that emulates the human brain’s neural network. Rather than simply running algorithms to completion, deep learning lets us tweak the parameters of a learning system until it outputs the results we desire. In 2019, the Turing Award, given for excellence in artificial intelligence research, was awarded to three of deep learning‘s most influential architects, Facebook’s Yann LeCun, Google’s Geoffrey Hinton, and professor Yoshua Bengio. This trio, along with many others over the past decade, developed the algorithms, systems, and techniques responsible for the onslaught of AI-powered products and services. (TNW)